Overview of Poisson Distribution and Normal Distribution
Statistics and data analysis often make use of Poisson distribution and normal probability distribution models as important statistical concepts.
Simeon-Denis Poisson first proposed his Poisson distribution in 1837 as an approach for modeling events occurring over a specific interval, such as time or space. With just one parameter representing average event rates and one parameter used as the measure of rarity of events like customers arriving per hour at shops or car accidents.
On particular roads daily – its use has grown immensely over time and today stands as testament to this principle’s power to accurately model rare but frequent happenings like customer arrival rates at stores or car accidents occurring every day on specific roads etc.
The Normal Distribution is also known as Gaussian Distribution and it is an analytical continuous probabilistic distribution often utilized in statistics. Carl Friedrich Gauss first proposed this distribution model during early nineteenth century; two parameters define its shape: mean represents center while standard deviation defines distribution spread – producing its characteristic bell-shaped curve for representing continuous data such as height, weight or IQ scores.
Poisson and normal distributions are both powerful probabilities distributions. Their differences stem from different ranges, shapes and applicability for various data. It’s essential that one understands these distinctions to select an apt distribution for any given data set and draw accurate statistical conclusions.
Understanding the difference between Poisson distribution and normal distribution is important
As it can have significant ramifications on decision making processes and analysis, understanding the differences between Poisson distributions and normal distributions is of vital importance for many reasons.
- Choosing the appropriate distribution: Accurate statistical analyses require precise distributions. Any deviation can lead to inaccurate conclusions and predictions. Understanding their differences will aid researchers in choosing an ideal distribution for their dataset.
- Modelling Different Data Types: Poisson Distribution is used for modeling count data while Normal Distribution can be applied to modeling continuous information. Understanding their differences allows researchers to choose which distribution best matches their dataset for more precise modelling and predictions.
- Understanding statistical results: Interpreting statistical analyses results can be complex without an in-depth knowledge of probability distributions and Poisson and normal distributions, so understanding their differences helps researchers interpret results more precisely and draw accurate conclusions from them.
- Communication of Results: Effective communication can be challenging when speaking to an audience with no prior statistical background, which makes understanding differences between distributions essential to communicating results more effectively and reaching wider audiences. Understanding them allows researchers to do this effectively and to improve results communication more broadly.
Understanding the differences between Poisson and normal distributions is vitally important in selecting an optimal distribution for any data set, modeling different forms of information and deciphering statistics.
Poisson Distribution
Poisson distributions are discrete probability distributions used to model how often events happen within any specified time or space interval. They were first proposed by French mathematician Simeon Denis in 1837 and have become widely adopted since.
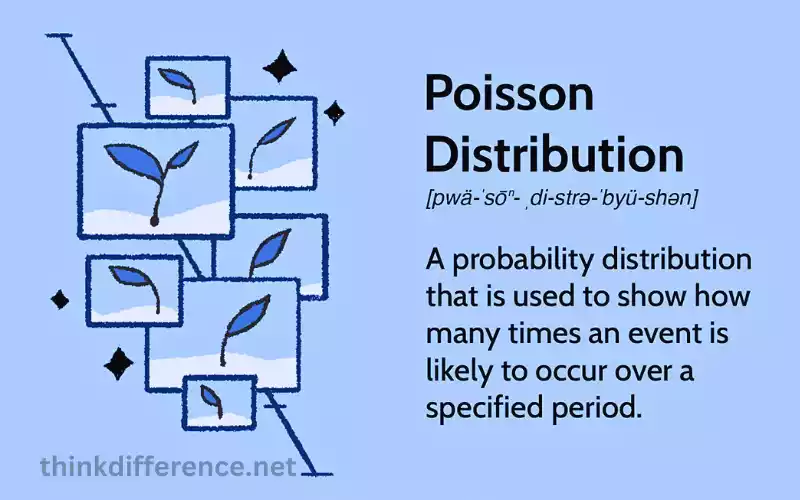
Poisson distribution only needs one parameter: lambda (l), representing the average rate of events. Below is the formula demonstrating its application as it will show how probable it is that at least k events occur in any time or space interval.
P(k) = (lk * e(-l))/k!
Euler’s number (2.71828) and K! the factorial are both calculated using this equation.
Poisson distributions possess various properties and traits, such as:
- Mean and variance: A Poisson distribution exhibits equal mean and variance values.
- Probability mass functions: To better illustrate Poisson distributions, probability mass functions (PMFs) provide an essential way of modeling it. They describe the probability that each value k will occur.
- Skewness and kurtosis: Skewness (skewness) and kurtosis are both positive. Poisson distribution has an excess of kurtosis with an expanded tail than normal distributions.
Poisson distributions have many uses in various fields of study and engineering applications:
- Counting events: Poisson Distribution Model of Events. When counting events, Poisson distribution can help model customer arrival rates at shops per hour or per day or number of car accidents on specific roads.
- Queuing theory: Poisson distributions can help queueing systems such as service centers or waiting lines predict customer arrival by using Poisson distributions to analyze arrival patterns.
- Reliability analysis: Reliability analysis relies heavily on Poisson distribution as an analysis method to calculate failure rates of mechanical or electronic components.
Poisson distribution is used to represent how often events happen in any time or space interval. It has three characteristic components – probability mass, skewness and kurtosis – and can be applied across many applications such as counting events or queuing theory.
Normal Distribution
The normal distribution, also referred to as Gaussian Distribution, is an ongoing probabilistic distribution commonly employed in data analysis and statistics. Carl Friedrich Gauss popularized its use in early nineteenth-century Germany.
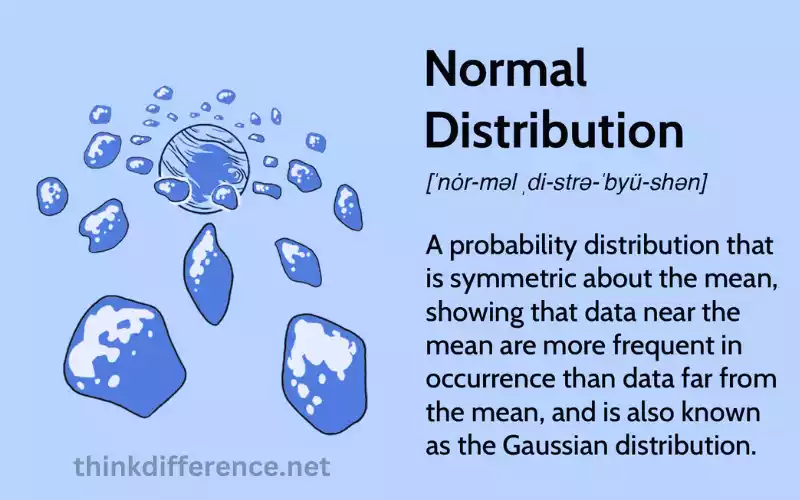
Normal distribution parameters consist of standard deviation (s) and mean (m). Here is the formula for its probability density function:
F(x) = (1/4 of (s*sqrt(2p))*e(-(x-m)2/2s2))
Where e is Euler’s number (approximately 2.71828), pi is the mathematical constant (approximately 31.4159), m stands for mean, s stands for standard deviation and sqrt represents square root function.
Normal distributions possess various properties and traits, including:
- Symmetry: Normal distributions feature an symmetrical mean with equal amounts of data on either side.
- Bell-shaped curve: Normal distribution curves take on the form of bell curves, with most data falling near to or at its median; as further data veers away from this position frequency decreases as far away it gets from it.
- Mean and variance: A normal distribution has both a mean and variance.
- Empirical Rule: The normal distribution follows an empirical rule which indicates that approximately 68% to 95% of data falls within one standard deviation from its mean; 99.7% fall within three standard deviations of this mean value.
Normal distribution can be applied across numerous disciplines:
- Inferential Statistics: Many inferential statistical tests such as the t test and ANOVA utilize normal distribution data to test hypotheses regarding means and variances.
- Quality control: Quality control uses normal distribution analysis to establish whether a process produces products within an acceptable range.
- Biostatistics: Biostatisticians often employ normal distribution as an accurate representation for many biological and physiological variables such as height and weight in biostatistics studies.
The normal distribution is an continuous probability distribution often used in data analysis and statistics. It has many characteristics such as symmetry, bell-shaped curves and empirical rules – many applications of which include inferential statistics, quality control as well as biostatistics.
Differences between Poisson and Normal Distributions
Poisson and Normal Distributions are two probabilistic distributions with similar properties and applications, their differences lie in their properties and application.
Here are their key distinctions:
- Type of Data: Poisson distributions are most frequently employed to represent discrete events like counts. Normal distributions provide continuous values within an envelope.
- Skewness: Positive skewness indicates a Poisson distribution, in which the right side tail of distributions has longer tails than left side tails. Normal distributions typically feature bell curves centered around their mean.
- Probability Density (PDF) of Poisson Distribution: Each possible count value assigned a probability; on the contrary, normal PDF’s assign probabilities based on ranges.
- Mean and Variance : For Poisson distributions, their Mean and Variance equal the parameter l that represents an event’s average rate at which it occurs; normal distribution’s Mean and Variance can take any value depending upon standard deviation and mean parameters.
- Applications: The Poisson Distribution is commonly used to represent discrete data such as daily accidents. Normal distributions can represent continuous information such as population height. Normal distributions also serve as useful hypothesis tests and statistical analysis tools while Poisson distributions tend to model reliability and queueing theory better.
Both Poisson distribution and normal distribution are important probability distributions; each has different properties and applications. Poisson can be used to model discrete count data with its discrete, positively skewed probability density function. While modeling continuous data using normal distribution’s bell-shaped probability function and its symmetrical shape is ideal for representing continuous information; its standard deviation and mean can take any value as shown below.
Conclusion
Poisson and Normal distributions are important probability distributions used in data analysis and statistics. While Poisson represents count data while normal covers continuous ones. Poisson features discrete probability functions with positive skewness while normal has continuous distributions with an almost symmetrical probability density function.
Understanding their differences is vital when selecting models to suit datasets effectively, conducting statistical analyses or inferencing processes, or making inferences from them.